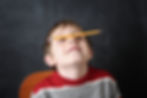
A neurofeedback protocol is a tailored, software-guided treatment approach to regulate brain activity. It employs real-time monitoring and biofeedback mechanisms to enhance or suppress neural oscillations in specific brain regions. A neurofeedback protocol seeks to normalize brain wave patterns to achieve a balanced neural state, boost neuroplasticity, improve mood, and alleviate symptoms related to neurological disorders (Hammond, 2011).
Approaches to training clients using neurofeedback vary widely but can be loosely divided into amplitude, deep states, slow cortical potential, infra-slow and ultra-low, EEG-guided, and qEEG-guided training. This post covers evidence-based protocols, how to select optimum protocols, and the importance of tailoring training to each client. It stresses that training a single EEG sensor site is not simply training that site alone but is likely engaging a wealth of cortical and subcortical resources to accomplish the task of causing the software display to change in the desired direction. Click on our narrator icon to listen to this post.
Amplitude Training
Amplitude training can use 1 or 2 sensor locations with training location and choice of training frequencies determined by client symptoms and the clinician’s knowledge of functional neuroanatomy and neurophysiology. A clinical EEG assessment may include the theta/beta ratio, eyes open and eyes closed alpha response, and the peak alpha frequency derived from 1-4 sensors and calculated by the clinician with reference to standard values from the field of electroencephalography.
The movie below is a 19-channel BioTrace+ /NeXus-32 display of SMR activity © John S. Anderson. Brighter colors represent higher SMR amplitudes. Frequency histograms are displayed for each channel. Notice the runs of high-amplitude SMR activity.
This training is often called amplitude and/or power training as it rewards either increases or decreases in the amplitude of the target frequencies. Training paradigms are often changed in response to client self-reports of symptom changes. Training usually involves working with multiple frequencies simultaneously, often in a reward/inhibit strategy (e.g., feedback is produced when one band, such as 12-15 Hz, has an amplitude above threshold, and the amplitudes of slower and faster bands are simultaneously below threshold).
Deep States Training
Deep states training is also a form of amplitude training. This usually involves training frequencies in the theta and alpha frequency bands, though it can also include slow cortical potentials. This training often has a more behavioral focus and is used for anxiety, panic, sleep, and other disorders, as well as PTSD and optimum performance.
Slow Cortical Potential Training
Slow cortical potential (SCP) training works with the cortical electrical gradient to facilitate either an increase or decrease in cortical excitability with the understanding that some pathological conditions, such as epilepsy or migraine, may be associated with excess excitability. In contrast, conditions like ADHD, inattentive type, may be associated with deficient cortical activation.
Infra-Slow and/or Ultra-Slow Training
Infra-low and/or ultra-slow training also uses an aspect of the cortical gradient to encourage regulation of this basic cortical measure, but they work in a domain with a very long time constant. Therefore, they function by giving the client feedback that varies as the cortical gradient signal varies, often with continuous, proportionally variable feedback (e.g., louder or softer sound based on higher or lower amplitude) of this signal in the form of visual and/or auditory information.
EEG-Guided Training
EEG-guided training requires a skilled electroencephalographer. This individual will evaluate the raw EEG and, because of their depth of experience interpreting the EEG, will train areas and frequencies determined by that analysis. An ongoing observation of the raw EEG will determine protocol changes during training.
qEEG-Guided Training
qEEG-guided training is guided by an analysis, often using a reference EEG normative database. Providers train brain areas with atypical activity toward statistically normal behavior EEG activity. Protocols are often performed with 1 or 2 scalp sensors, training individual locations identified by the qEEG. Protocol changes are generally determined by repeat qEEG assessments.
Z-Score Training
Z-score training is conducted using real-time comparisons of the client’s EEG with a normative database. Areas of dysregulation are trained toward typical values (i.e., Z = +/- 1.5 SD). A provider adapts the protocol based on client self-reports of symptom improvement or new concerns. This approach is usually guided by a 19-channel quantitative EEG (qEEG) assessment. Z-score protocols continuously record 2, 4, or 19 EEG channels and simultaneously train multiple variables.
The Evolution of Optimum Neurofeedback Protocols
Understanding some of the history of neurofeedback is very helpful to better appreciate the development of optimum protocols better. Neurofeedback training began with fairly simple, straightforward training approaches. For an in-depth discussion of neurofeedback, how it is administered, and more, please see Neurofeedback Tutor from BioSource Software.
Joe Kamiya studied volitional control of alpha frequency band (usually 8-12 Hz) amplitude with human participants (Kamiya 1970). Barry Sterman studied operant conditioning of EEG in cats (Sterman, 1974; Wyrwicka and & Sterman, 1968) and then rhesus monkeys (Sterman, Goodman, and & Kovalesky, 1978), identifying the approximately 14 Hz sensory motor rhythm (SMR – usually trained as 12-15 Hz) in the process. Sterman subsequently worked with humans with poorly regulated seizure conditions (Sterman & Friar, 1972; Sterman et al., 1974). This work was replicated by other researchers, showing continued positive results with refractory seizure participants (Lubar & Bahler, 1976; Seifert & Lubar, 1975). Sterman reviewed 24 studies with a total of 243 participants using this approach (Sterman, 2000), several including well-designed control conditions. This analysis showed that 82% of participants experienced improved seizure activity (decreased frequency and intensity) by 30% or more; the average improvement was more than 50%. This was later re-examined by Enger and Sterman (2006).
Early pioneers like Joel Lubar, Sue and Siegfried Othmer, Margaret Ayers, Michael Tansey, and others continued this work and developed new applications for different populations, such as children with ADHD. One common thread in much of the early work was a positive response from their clients, similar to that seen with the seizure studies.
The early training was almost exclusively performed using single EEG channels, using 3 electrodes including a positive, a negative, and a ground/reference. Sensor locations were often bi-polar montages (sensor configurations) where both the positive and negative sensors were on the scalp. Some were done with the positive sensor on a scalp location and the negative attached to a relatively neutral location like the earlobe. The ground/reference was always attached as well to complete the circuit.
Details of sensor location and placement, differential amplifiers, and how the EEG signal is derived can be found in Neurofeedback Tutor. There is also an extensive discussion of montage choices and their benefits and limitations in Nunez and Srinivasan (2006) for those wishing to explore this topic more fully.
Generally, training focused on a single EEG frequency band, such as 8-12 Hz (alpha) or 12-15 Hz (SMR). More complex training approaches emerged, such as simultaneously training to increase one frequency band while "inhibiting" one or two other bands. Much early training was done with children with attention deficit hyperactivity disorder (ADHD) (Lubar & Shouse, 1976). Clinicians reported that these children often benefited from increasing the amplitude of SMR or beta frequencies (15-18 Hz was a common beta frequency band). However, resourceful children soon discovered that simply clenching the jaw muscles caused the reward signal to increase (the author’s personal experience). Muscle electrical activity (EMG artifact) appears within the EEG frequencies and produces much faster frequencies, 1000 Hz and more (Rangayyan, 2015). Adding an inhibit filter allowed clinicians to set a threshold to prevent reward feedback to the client when the EMG artifact exceeded a predetermined value. This required the client to produce actual EEG changes rather than increasing muscle tension to trigger the reward.
Another common inhibit frequency was 4-8 Hz (theta) to discourage the kind of drifting attention typical of the ADHD inattentive diagnosis. Again, 4-8 Hz activity above the clinician’s set threshold inhibited the reward, reminding the client to remain attentive.
These reward and inhibit strategies followed operant conditioning procedures and involved discrete, event-related reinforcement. For example, when the training signal reached criteria, a reward was given, such as an auditory beep and/or a visual change on a computer display. The reward feedback was absent when the criteria were not met.
This training was often done with the positive, often called the active electrode, at the center midline scalp location known as Cz in the 10-20 international electrode placement system (the 10-20 system). This training approach was used at the New Visions School developed by A Chance to Grow in Minneapolis, MN (1992-1998, personal experience). The results were tracked by continuous performance tests (CPT), academic achievement, and behavioral reports from the student, teachers, support staff, and parents.
The chart below shows the results from 35 children, indicating the percentage of children in the typical (normal) range on the Test of Variables of Attention (T.O.V.A., Greenberg & Waldman, 1993), a type of CPT. Twenty-nine of the 35 students showed changes of at least 1 standard deviation from the initial testing results in at least one scale.
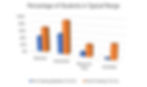
The chart above indicates the percentage of students taking the pre- and post-training T.O.V.A. in the typical range for the four scales. None of the students taking the initial pre-training baseline test were in the typical range on the variability scale. Following neurofeedback training, 40% were in the typical or normal range (Anderson, Program Evaluation, EEG Neurofeedback New Visions School – 1994-1995).
The second chart shows the percentage of students improving either 1 or 2 SD by the T.O.V.A. scale (Anderson, Program Evaluation, EEG Neurofeedback New Visions School – 1995-1996).
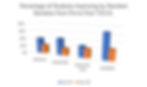
Subsequent years produced similar results, with increasing numbers of students receiving neurofeedback training each year.
These results were accomplished using single-channel training, often with a single Cz sensor location, generally following the work of Lubar and colleagues at the University of Tennessee (Lubar, 1991).
Later, following the work of Susan Othmer, other locations were added, and a common protocol evolved, training 15-18 Hz at the left side C3 location and 12-15 Hz at the right side C4 location, titrating the amount of time on either side depending on the student's level of hyperactivity or inattention. Generally, the more hyperactive students received increased training time at C4 using the 12-15 Hz reward band, while more inattentive students trained for longer periods at C3 using 15-18 Hz. This appeared to produce more targeted and specific training effects, activating the under-aroused students and calming the hyperactive students.
Other clinicians and researchers used a variety of training approaches. Michael Tansey used a custom-designed sensor that spanned the central midline sites of Fz, Cz, and Pz and trained for increases in 14 Hz., following Sterman’s research (Tansey, 1985; Tansey & Bruner, 1983), and demonstrated improvements in reading disorders, hyperactivity, mild neurological impairment and developmental delays (Tansey, 1990, 1991).
Joel Lubar and Vincent Monastra began to focus on ratio training between the 13-21 Hz beta band and the 4-7 Hz theta band, known as theta/beta ratio training at the Cz electrode location (Lubar, 1991). Monastra eventually published a study of 100 children diagnosed with ADHD with high theta/beta ratios measured at Cz before training (Monastra et al., 2002). ADHD indicators normalized, and medication was eliminated while maintaining the gains from training. A medication-only group deteriorated when medications were discontinued.
Joe Kamiya continued training individuals to control the alpha rhythm (Kamiya & Noles, 1970) and reduce anxiety (Hardt & Kamiya, 1978). This eventually led to alpha band training by researchers, including Barbara Brown (1974, 1977), Patricia Norris and Steve Fahrion (1990), Elmer and Alyce Green, Menninger Foundation colleagues (Passini et al., 1977), and Eugene Peniston.
Eugene Peniston studied at the Menninger Foundation and went on to utilize a modified version of alpha training that he dubbed alpha-theta training for patients in the inpatient alcohol treatment program at the Ft. Lyons Veterans Administration Hospital in Colorado (Peniston & Kulkosky, 1989, 1991). He trained alpha and theta frequencies at the Pz sensor location, providing feedback regarding both signals with the mild suggestion that increasing the number of theta beeps was desirable. This led to a brain activity state that became known as the crossover state, where theta exceeded alpha amplitude. The results were positive, with 8 of 10 participants in the EEG training group remaining abstinent at the 1-year follow-up. All 10 control group participants returned to active alcohol use and returned to inpatient treatment. In 1994, a much larger study was undertaken at a substance abuse treatment center in Los Angeles (Scott et al., 2005) with similar results, including on 3-year follow-up. Finally, a study of homeless Houston crack cocaine users also increased abstinence (Burkett et al., 2005).
This approach is currently in use in multiple treatment programs throughout the world. Eventually, clinicians began to use alpha-theta training with clients who presented with anxiety, early trauma, PTSD, and related concerns. It also became a common training approach for improving creativity and for optimum performance applications (Egner et al., 2002; Gruzelier, 2009; Raymond et al., 2005). Alpha-theta came to be known as deep states training. The Biofeedback Certification International Alliance (BCIA) offers several webinars that discuss this approach and its various applications on their website (https://bcia.mclms.net/en/package/list).
As relatively affordable EEG amplifiers became more available (Lexicor Medical Technology, Lexicor NRS-24, 1989), more clinicians began to use this technology to perform assessments. They often used the NYU normative database, more commonly known as Nx-Link, when combined with the Lexicor technology. This database, developed by E. Roy John and colleagues at New York University (John et al., 1977), fueled a revolution in identifying the locations of atypical activity in a client’s brain by recording 19 scalp electrode sites and comparing those findings to the normative database (Thatcher, 2010). This allowed clinicians to identify locations deviating from the values derived from the sample population of purportedly typical individuals, with comparisons based on age and eye condition (open or closed). From this, practitioners began up- and downtraining the atypical activity. If there were a deficit of posterior alpha in an individual with anxiety, providers would train clients to increase posterior alpha to normal levels (uptraining). If excess localized delta activity corresponded to an impact injury and client behavioral symptoms, they would train clients to decrease delta amplitude (downtraining). This allowed practitioners to target specific areas of abnormal activity rather than searching for a single best location or using a generic protocol.
Surmeli and colleagues (2015) used this approach to guide the training of 20 subjects diagnosed with dementia and showed significant improvement in outcome measures. Walker (2011) used this approach with 46 migraine patients, downtraining 21-30 Hz activity from 1-4 cortical areas, determined by qEEG assessment. Hammond (2003, 2004) followed a similar approach with clients experiencing obsessive-compulsive disorder (OCD) symptoms. The use of qEEG-guided assessment has become quite widespread, and many clinicians believe this is the best approach for determining training approaches and identifying optimum protocols.
Clinicians face several challenges using this approach. The learning curve for acquiring the qEEG assessment skills is quite steep. The clinician must become experienced in collecting a high-quality EEG recording, interpreting the raw EEG recording, identifying typical and atypical brain activity, and distinguishing it from the wide variety of artifacts that occur in virtually all EEG recordings. Next, the clinician must be able to select an adequate number of high-quality representative samples to be used for database comparison and analysis. Finally, the clinician must be able to interpret the database analysis results, which can run to several hundred pages of tables, graphs, brain maps, and other information (most practitioners of qEEG-guided neurofeedback, however, use more condensed analysis reports).
Alternatives to qEEG-Guided Neurofeedback
In addition to the task of learning how to perform an EEG analysis, the cost of equipment, software, and supplies is often over $2500 - $3000. This upfront investment may exceed the resources of new and even well-established clinicians. The prohibitive expense led to the proliferation of more accessible approaches. These protocols are based on more simplified evaluations, often using what has become known as a clinical database for the development of optimum protocols. These assessment tools utilize 1-8 sensors, often in a sequential manner, to record and analyze the client’s EEG, often during several behavioral conditions such as eyes open, eyes closed, mental math or reading tasks, physical movements, and more (Jarret et al., 2017; Soutar & Longo, 2022; Swingle, 2014). While these do not provide as comprehensive an assessment as a 19-channel recording with normative database comparison, many clinicians have reported positive training outcomes. Although we must cautiously evaluate anecdotal reports, the training is supported by a vast library of published efficacy research.
Another issue concerns the value of a 19-channel quantitative database assessment. Siegfried Othmer has articulated these concerns in numerous articles, papers, and book chapters. He addressed these limitations in his chapter on the history of neurofeedback in Restoring the Brain: Neurofeedback as an Integrative Approach to Health (Kirk, 2020).
Othmer has challenged moving from clinical assessment of client symptoms. He has emphasized the importance of understanding neurophysiology and behavioral neurology. Last, he has questioned the strategy of correcting deviations from the norm in the EEG itself, using database comparisons.
Although many experienced clinicians welcome an evidence-based approach utilizing normative databases to provide training prescriptions, this can discard clinical knowledge, experience, and insight that have informed successful neurofeedback training. Stories have emerged of clinicians continuing qEEG-guided training despite client self-reports of worsening symptoms (client self-reports, Hammond & Kirk, 2008). Othmer observes that some EEG findings of atypical activity represent compensatory behaviors, as the brain adjusts for anomalies in one area by increasing or decreasing activity in other areas. Training to eliminate atypical activity may result in symptoms or functional impairment.
A recent trend is only to train atypical activity when it corresponds to problematic symptoms reported by the client. For example, 19-channel surface and/or LORETA z-score training from Applied Neuroscience (NeuroGuide) uses a symptom checklist function to compare EEG findings to client symptoms. A clinician, hopefully with client input, selects a symptom or brain network to train. The software selects only those metrics within the client’s assessment that correspond to this selection and are statistically abnormal. Essentially, the software identifies CNS locations and metrics associated with a particular symptom and determines whether those locations and metrics are statistically abnormal. The clinician only trains these sites and metrics. The premise of z-score training is to train variables to Z = 0 (i.e., a standardized z-score represents the statistical average for a measure). For example, a practitioner can train delta-band amplitude at F4 toward zero standard deviations. This is the average for the same-aged participants who comprise the data. A clinician sets z-score thresholds for earning rewards.
Although this approach addresses one of Othmer's concerns, critics have questioned database validity. Their concerns include the database standardization sample (e.g., sample size, age, and biological sex), data collection methods (e.g., amplifiers, epoch length, and sampling rate), screening methods, and participant condition at the recording time. Factors like the quality of the previous night’s sleep, unreported alcohol or drug use, and unreported prior head injuries and illnesses can affect normative values. The growing body of published research supporting the efficacy of qEEG-guided training counters these potential concerns.
Another concern is whether the norm represents the optimum. Clinicians working with optimum-performance clients have goals that include helping clients become exceptional rather than typical. It may be that such z-score training is best reserved for individuals with clear pathologies. Some clinicians use z-score training to correct abnormal patterns and then use other approaches to encourage optimum results.
Slow cortical potential (SCP) training emerged primarily from the work of Neils Birbaumer and Ute Strehl (Birbaumer et al., 1990; Kotchoubey et al., 2001; Mayer et al., 2012; Rockstroh et al., 1993; Strehl, 2006). This training is associated with the contingent negative variation (CNV), a component of the event-related potential (ERP) that reflects shifts in cortical electrical gradients from positive to negative. These shifts are most commonly observed during a go/no-go task (Luck, 2014). Sarah Wyckoff (2013) wrote an excellent article on SCP neurofeedback training in ISNR's NeuroConnections Newsletter special edition on the various slow cortical, infra-low, and infra-slow approaches. This training has been used for epilepsy, migraine, and ADHD. Edith Schneider (2013) provided clinical descriptions and examples in the same issue, with special emphasis on childhood trauma and PTSD.
Infra-low training, introduced by Sue and Siegfried Othmer, generated much controversy within the neurofeedback community, likely due to misconceptions about the nature of the training. This was further exacerbated by the lack of concrete explanations of what was being done, what frequencies were being trained, and how the signal was processed (Siegfried Othmer, personal communication). In his chapter on the history of neurofeedback, Othmer (2020) explained that the training signal is related to signals as low as 0.0001 Hz or 0.1 millihertz (0.1 mHz). He described the hypothetical training mechanisms and offered analogies to the resting state or default mode networks and their interactions with executive networks, mediated by the salience network. He also described the training not as operant conditioning but as providing a constantly varying signal to the client and allowing their brain to use this information to self-regulate. Hopefully, the large base of practitioners using this approach will produce studies helping to quantify the effectiveness of this approach. Several anecdotal reports suggest that this may be a very powerful intervention.
Following the introduction of infra-low training by the Othmers, Mark Smith began working with what he terms infra-slow (ISF) EEG training. He distinguished between activity in the 0.01-0.1 Hz range and that occurring below 0.01 and between DC shifts and the ISF signal (Smith, 2013). These distinctions relate to voltage and frequency differences, with DC shifts occurring in the millivolt (mV) range and ISF fluctuations occurring in the microvolt range (µV). He also utilizes qEEG assessment and various inhibit strategies in conjunction with the ISF training.
Smith summarized the findings of ISF signal studies from various researchers (Keković et al., 2012; Nir et al., 2008; Palva & Palva, 2012; Pfurtscheller et al., 2012; Vanhatalo et al., 2004; Zschocke & Speckmann, 1993) citing various descriptions of the ISF signal and physiological measures that may be associated with it. Other researchers have published on this method, including randomized, controlled trials using sham feedback (Bazzana et al., 2022; Mathew et al., 2022). David Kaiser (2013) provided an excellent overview of the likely mechanisms underlying ISF signals, especially the glial system. For those interested in this area of study, this issue of NeuroConnections is highly recommended.
Those who have made it this far into this post might wonder how we will resolve the question of finding the optimum neurofeedback protocol.
Competing Perspectives
Some neurofeedback providers emphasize structured protocols guided by well-defined parameters. This group utilizes operant conditioning, sometimes to the point of complex reward strategies that allow for the post-reinforcement synchronization identified by Sterman and colleagues and others (Clemente, Sterman, & Wyrwicka, 1964), and other optimal learning components.
A separate camp (Anderson, 2022; Othmer, 2016; Smith, 2013) advocates providing information to the client so that their brain can use it to self-correct. These practitioners provide visual or auditory feedback to the client that represents the information derived from the EEG, usually as an ongoing, continuously varying signal.
This feedback approach can represent almost any physiological measure, from infra-low and infra-slow oscillations to the amplitude fluctuations common to amplitude training to z-score training, as the signal varies toward or away from the goal of zero standard deviations. The continuous feedback signal to the client is proportional to the value represented, either as volume increases or decreases or as visual display changes. A vertical or horizontal bar graph illustrates proportional feedback. It fluctuates up and down or side to side in response to the represented signal. This type of feedback display is seen in many neurofeedback training screens from multiple software systems and represents constant proportional feedback if it is visible to the client. Unbeknownst to many practitioners who claim to be only doing discrete, event-related feedback, this common display may also provide continuous proportional feedback simultaneously and help explain the historical success of neurofeedback training.
Research into the use of discrete, event-related feedback (exceeding a set threshold produces feedback) or continuous feedback yields interesting results in brain-computer interface studies (Carino-Escobar et al., 2023). Brain-computer interface analysis showed improved performance with continuous feedback compared with discrete feedback in 12 of 18 participants. The researchers suggest this was due to “enhancement of attentional and closed-loop somatosensory processes,” similar to that suggested by Othmer (2016).
Congedo, Lubar, and Joffe (2004) used a combination of discrete and continuous feedback in their study of LORETA neurofeedback. Vernon (2003) suggests that a combination of discrete and continuous feedback was optimal for training SMR amplitude in their study participants. Fokina and colleagues (2010) showed that continuous proportional audio feedback led to changes in the theta/beta ratio in cats trained with a varying tone that represented increases and decreases in that ratio, demonstrating the effectiveness of this approach.
Perez-Elvira and colleagues (2021) also note the importance of motivational value when choosing feedback. They found that allowing the client/participant to select the reinforcement significantly improved success when training for increased sensorimotor rhythm amplitude in a study of 113 individuals randomly assigned to multiple conditions, including a sham feedback control. Involving the client in selecting the feedback display (visual and/or auditory, discrete, continuous, or combined) may enhance training outcomes.
Optimum Protocol Selection Is A Process
Scientific research often compares treatment protocol efficacy for treating identified groups (e.g., ADHD, inattentive subtype). Although these studies can identify candidate protocols, clinicians must select optimal protocols for individual clients. Like medication prescription, selecting an optimum neurofeedback protocol is a continuous process rather than a one-time event. A clinician may select an evidence-based neurofeedback protocol guided by client preference, provider experience, and resource availability. They must adjust this protocol based on measured outcomes (e.g., EEG parameters, symptoms, and performance) and the client's changing goals.
Protocol selection depends on practical considerations, client preferences, and cultural values. Among practical factors are access to equipment and software, practitioner expertise, financial constraints, and physical distance from the clinic. Another concern is the client's willingness to participate in multiple-sensor training, including messy electrode caps requiring gel injection into individual electrodes. Although dry electrodes have addressed this concern, they are often more expensive. Children may dislike the restrictive nature of the cap systems and be unwilling to participate in ongoing training. Individuals who wear head coverings as a religious or cultural observance may not allow their heads to be uncovered. They may require a clinician of a particular gender (usually female), limiting the ability of these clients to participate in the training.
Is there a "best" neurofeedback training approach? That question will likely remain unanswered for the foreseeable future. Individuals making these claims must "show their receipts," backing up their assertions with peer-reviewed scientific evidence.
A Practitioner's Journey from Entry-Level Knowledge to Expertise
Real-time neurofeedback training results in client learning, which can cause negative or positive effects. Providers require the expertise to adjust treatment protocols to minimize the negative and maximize the positive effects. They can gain this expertise through in-person, virtual, and hybrid training programs, personal training and non-clinical practice with volunteers, mentoring, and advanced workshops. Biofeedback Certification International Alliance (BCIA) certification and recertification involves these educational components. Applicants initially gain entry-level knowledge and then build on this foundation by training clients, reading the literature, and attending professional conferences, webinars, and workshops. Initially, new practitioners may utilize relatively simple approaches with limited customization. As they gain experience, they will add more tools to their toolkits, applying different approaches to clients based on their unique needs, individual differences, and abilities. This results in the flexible, tailored, unique intervention that best fits the client and is the optimum training protocol for that person. The optimum training protocol evolves with the provider’s growing knowledge, skill, and availability of hardware/software resources. Finally, training any location on the scalp involves many interactive neural networks (Hellurng et al., 2022; Mirifar et al., 2022; Ninaus et al., 2013). Rewarding an increase in SMR at the Cz location doesn’t just involve neurons immediately below the sensor. Making that specific change in the actively recorded neurons must involve many more systems (Cera et al., 2019). This must include visual and auditory networks, which receive, process, and interpret visual and auditory feedback provided by the training software. Then, the executive networks direct efforts to develop volitional control over the feedback display. This must also involve interactions between task-positive and task-negative networks and include the salience network, which determines the importance of internal and external sensory inputs and directs attentional resources toward the important ones.
Therefore, training a single EEG sensor site is not simply training that site alone but is likely engaging a wealth of cortical and subcortical resources to accomplish the task of causing the software display to change in the desired direction. This may ultimately be the single factor common to all neurofeedback training approaches – training the brain by providing accurate, timely, and useful information about its functions appears to allow the brain to self-correct in a way that can be highly therapeutic and significantly improve brain function.
Quiz
Take a five-question quiz on Quiz Maker to assess your mastery.
Glossary
amplitude training: neurofeedback protocols that target the enhancement or reduction of specific EEG frequency amplitudes. Attention Deficit Hyperactivity Disorder (ADHD): a neurodevelopmental disorder characterized by persistent patterns of inattention, hyperactivity, and impulsivity that interfere with functioning or development.
deep states training: training deeper states of consciousness, often achieved through low-frequency brain wave entrainment.
downtraining: decreasing the amplitude or power of specific EEG frequency bands. dry electrodes: sensors that detect the EEG without conductive gels or solutions, often used in portable or wearable EEG systems.
EEG-guided training: protocols utilizing real-time EEG data to guide neurofeedback training. infra-low training: neurofeedback protocols that target oscillations as low as 0.0001 Hz.
infra-slow training: neurofeedback protocols that target infra-slow oscillations.
low-resolution electromagnetic tomography (LORETA): Pascual-Marqui's mathematical inverse solution to identify the cortical sources of 19-electrode quantitative data acquired from the scalp. neurofeedback protocol: a tailored, software-guided treatment approach to regulate brain activity. It employs real-time monitoring and biofeedback mechanisms to enhance or suppress neural oscillations in specific brain regions. power: the amplitude squared and may be expressed as microvolts squared or picowatts/resistance.
qEEG-guided training: neurofeedback protocols using 19 or more channels, often referenced to a quantitative EEG (qEEG) database.
ratio training: neurofeedback protocols that adjust the ratio between different frequency bands to achieve a specific neurophysiological or cognitive outcome.
sensorimotor rhythm (SMR): the 13-15 Hz spindle-shaped sensorimotor rhythm (SMR) is detected from the sensorimotor strip when individuals reduce attention to sensory input and reduce motor activity.
slow cortical potential (SCP) training: neurofeedback protocols that target slow cortical potentials to improve self-regulation of brain activity.
ultra-slow training: neurofeedback protocols that target ultra-slow oscillations, generally slower than infra-slow oscillations, for regulating deeper neurological and physiological states.
uptraining: neurofeedback to increase the amplitude or power of specific EEG frequency bands.
z-score training: neurofeedback protocols that reinforce in real-time closer approximations of client EEG values to those in a normative database.
theta-to-beta ratio: the ratio between 4-7 Hz theta and 13-21 Hz beta, measured most typically along the midline and generally in the anterior midline near the 10-20 system location Fz.
References
Bazzana, F., Finzi, S., Di Fini, G., & Veglia, F. (2022). Infra-low frequency neurofeedback: A systematic mixed studies review. Frontiers in Human Neuroscience, 16, 920659. https://doi.org/10.3389/fnhum.2022.920659
Burkett, S. V., Cummins, J. M., Dickson, R., & Skolnick, M. H. (2005). An open clinical trial utilizing real-time EEG operant conditioning as an adjunctive therapy in the treatment of crack cocaine dependence. Journal of Neurotherapy, 9(2), 27–47. https://doi.org/10.1300/J184v09n02_03
Carino-Escobar, R. I., Rodríguez-García, M. E., Carrillo-Mora, P., Valdés-Cristerna, R., & Cantillo-Negrete, J. (2023). Continuous versus discrete robotic feedback for brain-computer interfaces aimed for neurorehabilitation. Frontiers in Neurorobotics, 17, 1015464. https://doi.org/ 0.3389/fnbot.2023.1015464. PMID: 36925628; PMCID: PMC10011154.
Cera, N., Esposito, R., Cieri, F., & Tartaro, A. (2019). Altered cingulate cortex functional connectivity in normal aging and mild cognitive impairment. Frontiers in Neuroscience, 13, 857. https://doi.org/ 10.3389/fnins.2019.00857
Clemente, C. D., Sterman, M.B., & Wyrwicka, W. (1964). Post-reinforcement eeg synchronization during alimentary behavior. Electroencephalography and Clinical Neurophysiology, 16, 355-65. https://doi.org/10.1016/0013-4694(64)90069-0. PMID: 14141754.
Egner, T., Strawson, E., & Gruzelier, J. H. (2002). EEG signature and phenomenology of alpha/theta neurofeedback training versus mock feedback. Applied Psychophysiology and Biofeedback, 27(4), 261-70. https://doi.org/10.1023/a:1021063416558
Fahrion, S. L., & Norris, P. A. (1990). Bulletin of the Menninger Clinic, 54(2), 217.
Fokina, Y. O., Kulichenko, A. M. & Pavlenko, V. B. (2010). Changes in the power levels of cortical EEG rhythms in cats during training using acoustic feedback signals. Neuroscience and Behavioral Physiology, 40, 951–954.
Greenberg, L. M., & Waldman, I. D. (1993). Developmental normative data on the test of variables of attention (T.O.V.A.). Journal of Child Psychology and Psychiatry, 34(6), 1019-30. https://doi.org/10.1111/j.1469-7610.1993.tb01105.x
Gruzelier, J. (2009). A theory of alpha/theta neurofeedback, creative performance enhancement, long distance functional connectivity and psychological integration. Cognitive Processing, 10 Suppl 1, S101–S109. https://doi.org/10.1007/s10339-008-0248-5.
Hammond, D. C. (2003). QEEG-guided neurofeedback in the treatment of obsessive compulsive disorder. Journal of Neurotherapy, 7(2), 25–52. https://doi.org/10.1300/J184v07n02_03
Hammond, D. C. (2004). Treatment of the obsessional subtype of obsessive compulsive disorder with neurofeedback. Biofeedback, 32, 9–12. Hammond, D. C. (2011). What is neurofeedback? An update. Journal of Neurotherapy, 15(4). https://doi.org/10.1080/10874208.2011.623090
Hammond C., & Kirk, L., (2008). First, do no harm. Adverse effects and the need for practice standards in neurofeedback. Journal of Neurotherapy, 12(1), 79-88. https://doi.org/10.1080/10874200802219947
Hardt, J. V., & Kamiya, J. (1978). Anxiety change through electroencephalographic alpha feedback seen only in high anxiety subjects. Science, 201(4350), 79–81. https://doi.org/10.1126/science.663641
Hellrung, L., Kirschner, M., Sulzer, J., Sladky, R., Scharnowski, F., Herdener, M., &Tobler, P. N., (2022). Analysis of individual differences in neurofeedback training illuminates successful self-regulation of the dopaminergic midbrain. Communications Biology, 5(1), 845. https://doi.org/10.1038/s42003-022-03756-4. PMID: 35986202; PMCID: PMC9391365.
Howe, R. C., & Sterman, M. B. (1972). Cortical-subcortical EEG correlates of suppressed motor behavior during sleep and waking in the cat. Electroencephalography and Clinical Neurophysiology, 32(6), 681–695. https://doi.org/10.1016/0013-4694(72)90104-6
Jarrett, M. A., Gable, P. A., Rondon, A. T., Neal, L. B., Price, H. F., & Hilton, D. C. (2020). An EEG study of children with and without ADHD symptoms: Between-group differences and associations with sluggish cognitive tempo symptoms. Journal of Attention Disorders, 24(7), 1002-1010. https://doi.org/10.1177/1087054717723986
John, E. R., Ahn, H., Prichep, L., Trepetin, M., Brown, D., & Kaye, H. (1980). Developmental equations for the electroencephalogram. Science, 210(4475), 1255-1258. https://doi.org/10.1126/science.7434026
Kaiser, D. (2013). NeuroConnections, Newsletter of the International Society of Neuroregulation and Research (ISNR).
Keković, G., Sekulic, S., Podgorac, J., Podgorac, & Bukurov, K. G. (2012). The slow and infraslow oscillations of cortical neural network. Neurology, Psychiatry and Brain Research, 18(4), 175–180. https://doi.org/10.1016/j.npbr.2012.04.003
Kirk, H. W. (Ed.). (2016). Restoring the brain: Neurofeedback as an integrative approach to health. CRC Press, Taylor and Francis Group.
Lubar J. F. (1991). Discourse on the development of EEG diagnostics and biofeedback for attention-deficit/hyperactivity disorders. Biofeedback and Self-Regulation, 16(3), 201–225. https://doi.org/10.1007/BF01000016
Lubar, J. F., & Bahler, W. W. (1976). Behavioral management of epileptic seizures following EEG biofeedback training of the sensorimotor rhythm. Biofeedback and Self-Regulation, 1(1), 77–104. https://doi.org/10.1007/BF00998692
Lubar, J. F., & Shouse, M. N. (1976). EEG and behavioral changes in a hyperkinetic child concurrent with training of the sensorimotor rhythm (SMR): A preliminary report. Biofeedback and Self-Regulation, 1(3), 293–306. https://doi.org/10.1007/BF01001170
Mathew, J., Adhia, D. B., Smith, M. L., De Ridder, D., & Mani, R. (2022). Source localized infraslow neurofeedback training in people with chronic painful knee osteoarthritis: A randomized, double-blind, sham-controlled feasibility clinical trial. Frontiers in Neuroscience, 16, 899772. https://doi.org/10.3389/fnins.2022.899772
Mirifar, A., Keil, A., & Ehrlenspiel, F. (2022). Neurofeedback and neural self-regulation: A new perspective based on allostasis. Reviews in the Neurosciences, 33(6), 607–629. https://doi.org/10.1515/revneuro-2021-0133
Monastra, V. J., Monastra, D. M., & George, S. (2002). The effects of stimulant therapy, EEG biofeedback, and parenting style on the primary symptoms of attention-deficit/hyperactivity disorder. Applied Psychophysiology and Biofeedback, 27(4), 231–249. https://doi.org/10.1023/a:1021018700609
Ninaus, M., Kober, S. E., Witte, M., Koschutnig, K., Stangl, M., Neuper, C., & Wood, G. (2013). Neural substrates of cognitive control under the belief of getting neurofeedback training. Frontiers in Human Neuroscience, 7, 914. https://doi.org/10.3389/fnhum.2013.00914
Nir, Y., Mukamel, R., Dinstein, I., Privman, E., Harel, M., Fisch, L., Gelbard-Sagiv, H., Kipervasser, S., Andelman, F., Neufeld, M. Y., Kramer, U., Arieli, A., Fried, I., & Malach, R. (2008). Interhemispheric correlations of slow spontaneous neuronal fluctuations revealed in human sensory cortex. Nature Neuroscience, 11(9), 1100–1108. https://doi.org/10.1038/nn.2177
Nowlis, D. P., & Kamiya, J. (1970). The control of electroencephalographic alpha rhythms through auditory feedback and the associated mental activity. Psychophysiology, 6(4), 476–484. https://doi.org/10.1111/j.1469-8986.1970.tb01756.x
Nunez, P. L., & Srinivasan, R. (Eds.). (2006). Electric fields of the brain: The neurophysics of EEG (2nd ed.). Oxford University Press.
Palva, S., & Palva, J. M. (2012). Discovering oscillatory interaction networks with M/EEG: Challenges and breakthroughs. Trends in Cognitive Sciences, 16(4), 219–230. https://doi.org/10.1016/j.tics.2012.02.004
Peniston, E. G., & Kulkosky, P. J. (1989). Alpha-theta brainwave training and beta-endorphin levels in alcoholics. Alcoholism, Clinical and Experimental Research, 13(2), 271–279. https://doi.org/10.1111/j.1530-0277.1989.tb00325.x
Peniston, E. G., & Kulkosky, P. J. (1991). Alpha-theta brainwave neuro-feedback therapy for Vietnam veterans with combat-related post-traumatic stress disorder. Medical Psychotherapy: An International Journal, 4, 47–60
Pérez-Elvira, R., Oltra-Cucarella, J., Carrobles, J. A., Moltó, J., Flórez, M., Parra, S., Agudo, M., Saez, C., Guarino, S., Costea, R. M., & Neamtu, B. (2021). Enhancing the effects of neurofeedback training: The Motivational value of the reinforcers. Brain Sciences, 11(4), 457. https://doi.org/10.3390/brainsci11040457
Pfurtscheller, G., Daly, I., Bauernfeind, G., & Müller-Putz, G. R. (2012). Coupling between intrinsic prefrontal HbO2 and central EEG beta power oscillations in the resting brain. PloS one, 7(8), e43640. https://doi.org/10.1371/journal.pone.0043640
Rangayyan, R. M. (2015). Biomedical signal analysis (2nd ed.). IEEE Press Series in Biomedical Engineering, IEEE Press.
Raymond, J., Varney, C., Parkinson, L. A., & Gruzelier, J. H. (2005). The effects of alpha/theta neurofeedback on personality and mood. Brain research. Cognitive Brain Research, 23(2-3), 287–292. https://doi.org/10.1016/j.cogbrainres.2004.10.023
Scott, W. C., Kaiser, D., Othmer, S., & Sideroff, S. I. (2005). Effects of an EEG biofeedback protocol on a mixed substance abusing population. The American Journal of Drug and Alcohol Abuse, 31(3), 455–469. https://doi.org/10.1081/ada-200056807
Seifert, A. R., & Lubar, J. F. (1975). Reduction of epileptic seizures through EEG biofeedback training. Biological Psychology, 3(3), 157–184. https://doi.org/10.1016/0301-0511(75)90033-2
Soutar, R., & Longo, R. (2022). Doing neurofeedback: An introduction (2nd ed.). Foundation for Neurofeedback and Neuromodulation Research
Sterman, M. B. (1976). Effects of brain surgery and EEG operant conditioning on seizure latency following monomethylhydrazine intoxication in the cat. Experimental Neurology, 50(3), 757–765. https://doi.org/10.1016/0014-4886(76)90041-8
Sterman, M. B. (2000). Basic concepts and clinical findings in the treatment of seizure disorders with EEG operant conditioning. Clinical EEG (Electroencephalography), 31(1), 45–55. https://doi.org/10.1177/155005940003100111
Sterman, M. B., Goodman, S. J., & Kovalesky, R. A. (1978). Effects of sensorimotor EEG feedback training on seizure susceptibility in the rhesus monkey. Experimental Neurology, 62(3), 735–747. https://doi.org/10.1016/0014-4886(78)90281-9
Sterman, M. B., Macdonald, L. R., & Stone, R. K. (1974). Biofeedback training of the sensorimotor electroencephalogram rhythm in man: Effects on epilepsy. Epilepsia, 15(3), 395–416. https://doi.org/10.1111/j.1528-1157.1974.tb04016.x
Strehl, U., Leins, U., Goth, G., Klinger, C., Hinterberger, T., & Birbaumer, N. (2006). Self-regulation of slow cortical potentials: A new treatment for children with attention-deficit/hyperactivity disorder. Pediatrics, 118(5), e1530–e1540. https://doi.org/10.1542/peds.2005-2478
Surmeli, T., Eralp, E., Mustafazade, I., Kos, H., Özer, G. E., & Surmeli, O. H. (2016). Quantitative EEG neurometric analysis-guided neurofeedback treatment in dementia: 20 Cases. How neurometric analysis is important for the treatment of dementia and as a biomarker?Clinical EEG and Neuroscience, 47(2), 118–133. https://doi.org/10.1177/1550059415590750
Tansey, M. A. (1990). Righting the rhythms of reason: EEG biofeedback training as a therapeutic modality in a clinical office setting. Medical Psychotherapy, 3, 57-68.
Tansey, M. A. (1991). Wechsler (WISC-R) changes following treatment of learning disabilities via EEG biofeedback training in a private practice setting. Australian Journal of Psychology, 43, 147-153.
Thatcher, R. W. (2010). Validity and reliability of quantitative electroencephalography. Journal of Neurotherapy, 14(2), 122-152. https://doi.org/10.1080/10874201003773500
Vanhatalo, S., Palva, J. M., Holmes, M. D., Miller, J. W., Voipio, J., & Kaila, K. (2004). Infraslow oscillations modulate excitability and interictal epileptic activity in the human cortex during sleep. Proceedings of the National Academy of Sciences, 101(14),5053-5057. https://doi.org/10.1073/pnas.0305375101
Vernon, D., Egner, T., Cooper, N., Compton, T., Neilands, C., Sheri, A., & Gruzelier, J. (2003). The effect of training distinct neurofeedback protocols on aspects of cognitive performance. International Journal of Psychophysiology: Official Journal of the International Organization of Psychophysiology, 47(1), 75–85. https://doi.org/10.1016/s0167-8760(02)00091-0
Walker J. E. (2011). QEEG-guided neurofeedback for recurrent migraine headaches. Clinical EEG and Neuroscience, 42(1), 59–61. https://doi.org/10.1177/155005941104200112
Wyckoff, S. (2013). NeuroConnections, Newsletter of the International Society of Neuroregulation and Research (ISNR).
Zschocke, S., & Speckmann, E.-J. (2012). Basic mechanisms of the EEG. Springer.
Learn More
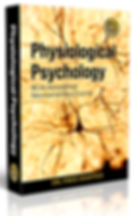